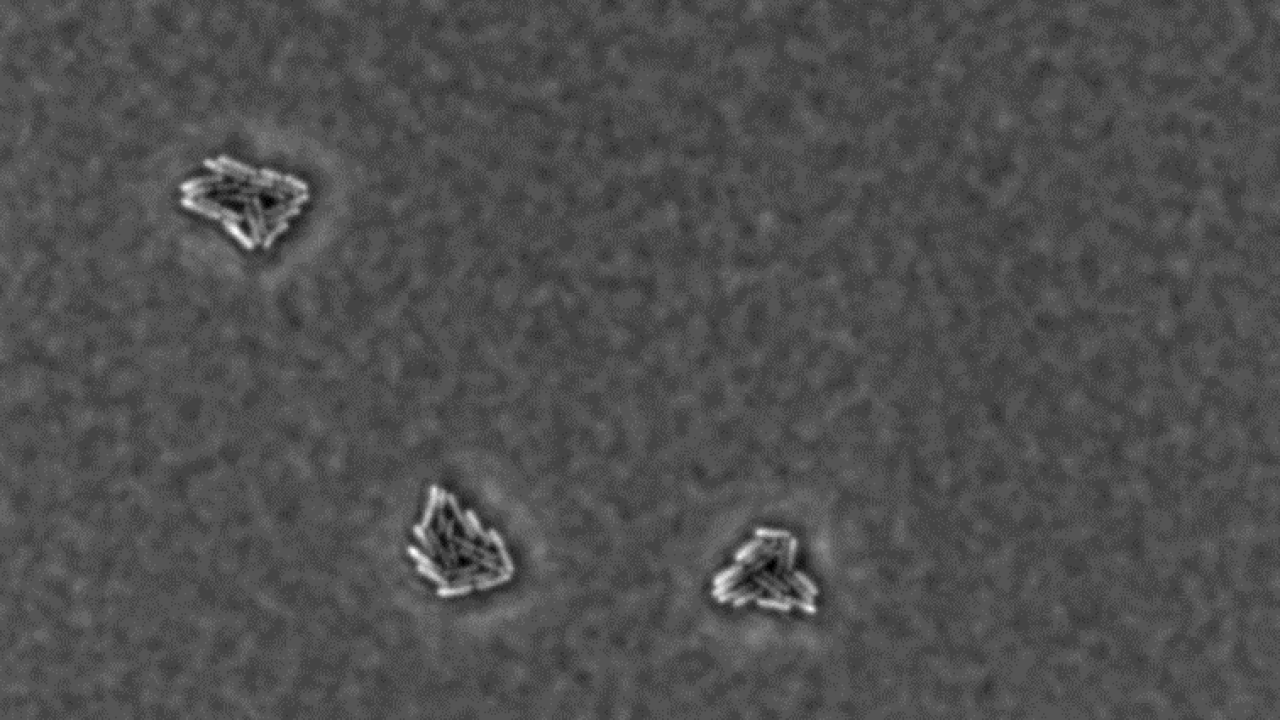
Researchers Develop Method for Rapid Bacteria Detection in Food
Artificial intelligence could be a valuable tool for the future of food safety. New research out of the University of California, Davis, finds that a technique using AI and optical imaging can quickly and accurately identify bacteria in food, making it a promising approach for preventing foodborne outbreaks and illnesses.
The study, published by journal Applied and Environmental Microbiology, finds using an algorithm called You Only Look Once (YOLO, version 4) to detect bacteria is a simple, cost-effective and rapid approach compared to conventional pathogen detection methods. The team of interdisciplinary researchers took digital images of romaine lettuce with a conventional microscope equipped with white light and used AI-enabled software to detect and identify bacterial microcolonies.
When put to the test, researchers said the real-time object detection algorithm accurately identified 11 out of 12 lettuce samples that were contaminated with E. coli.
Visual of how YOLO detects and identifies bacteria in microscopic image
Time-saving technology
Researchers said this AI-assisted detection has another advantage – its high speed. Luyao Ma, lead author and postdoctoral researcher with the Department of Food Science and Technology, said this technology can finish analysis within three hours, while conventional pathogen detection methods that rely on microbial culture can take several days before getting results.
“Once the food is processed, it can be tested and, on the same day, you can make sure there’s no pathogens and distribute it,” Ma said. “That is a very rapid screening.”
Ma said YOLO is commonly used in several real-world applications, including autonomous cars. She said they wanted to determine if the machine learning that identifies objects like cars or traffic lights can similarly identify different bacteria on a single microscopic image of a food item.
“People are using this algorithm to change people’s lives,” Ma said. “As food scientists, we can bring this knowledge into the agricultural food system.”
Telling bacteria apart
Nitin Nitin, professor with both food science and technology and biological and agricultural engineering, said the study also analyzed if the AI-enabled approach was capable of differentiating features of various bacteria. They found that YOLO discriminated E. coli from seven other common foodborne bacterial species, including Salmonella, with an average precision of 94%.
“With YOLO, we were able to both detect where these microcolonies are spatially located and identify which microorganism they belong to, and it was powerful enough to even differentiate among different strains of E. coli within that matter,” Nitin said.
He hopes their findings can help provide a framework to strengthen the ability to detect bacteria that can lead to outbreaks. There’s also potential to create automated food safety inspections using this AI method.
“With this advancement, we can deliver an even higher level of safety,” Nitin said. “Food safety is an essential part of food business, so if we can strengthen that area by providing a cost-effective, rapid, highly-sensitive and specific approach, I think the method, overall, should give consumers more confidence in our food systems as we move forward.”
Other authors of the study are Mason Earles with the Department of Viticulture and Enology, Nicharee Wisuthiphaet with the Department of Food Science and Technology and Jiyoon Yi, who was with the Department of Biological and Agricultural Engineering during the study.
Media Resources
- Luyao Ma, Department of Food Science and Technology, luma@ucdavis.edu
- Nitin Nitin, Department of Food Science and Technology and Department of Biological and Agricultural Engineering, nnitin@ucdavis.edu
- Tiffany Dobbyn, College of Agricultural and Environmental Sciences, tadobbyn@ucdavis.edu